Blending Problem#
This problem is taken from PuLP case studies.
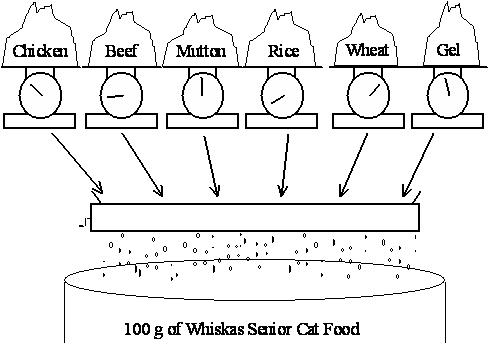
This example shows some basic usage of OptFlow similar to other modeling languages (CVXPY, PuLP, Pyomo, etc.), such as flow.Variable
, flow.Input
and flow.Constant
, and how NumPy arrays np.ndarray
can be used as constants in OptFlow.
# Author: Anonymized for paper review
# Case Study: The Blending problem
# https://coin-or.github.io/pulp/CaseStudies/a_blending_problem.html
import optflow as flow
x_1 = flow.Variable(flow.continuous)
x_2 = flow.Variable(flow.continuous)
obj = 0.013 * x_1 + 0.008 * x_2
constraints = [
x_1 + x_2 == 100.0,
0.1 * x_1 + 0.2 * x_2 >= 8.0,
0.08 * x_1 + 0.1 * x_2 >= 6.0,
0.001 * x_1 + 0.005 * x_2 <= 5.0,
0.002 * x_1 + 0.005 * x_2 <= 0.4
]
prob = flow.Problem(objective=obj, constraints=constraints, sense=flow.minimize)
opt_obj = prob.solve(solver=flow.programming)
print(opt_obj)
print(x_1.optimized_value)
print(x_2.optimized_value)
# Author: Anonymized for paper review
# Case Study: The Blending problem
# https://coin-or.github.io/pulp/CaseStudies/a_blending_problem.html
import optflow as flow
import numpy as np
N = 2
x = flow.Variable(flow.continuous, shape=(N, ))
cost = flow.Input(dtype=flow.float64, shape=(N, ))
percentage = flow.Constant(dtype=flow.float64, shape=(4, N),
value=np.array([[0.1, 0.2], [0.08, 0.1], [0.001, 0.005], [0.002, 0.005]]))
obj = sum(cost[i] * x[i] for i in range(N))
constraints = [
x[0] + x[1] == 100.0,
percentage[0, 0] * x[0] + percentage[0, 1] * x[1] >= 8.0,
percentage[1, 0] * x[0] + percentage[1, 1] * x[1] >= 6.0,
percentage[2, 0] * x[0] + percentage[2, 1] * x[1] <= 5.0,
percentage[3, 0] * x[0] + percentage[3, 1] * x[1] <= 0.4
]
prob = flow.Problem(objective=obj, constraints=constraints, sense=flow.minimize)
opt_obj = prob.solve(solver=flow.programming, inputs={cost: np.array([0.013, 0.008])})
print(opt_obj)
print(x[0].optimized_value)
print(x[1].optimized_value)
# Author: Anonymized for paper review
# Case Study: The Blending problem (with input)
# https://coin-or.github.io/pulp/CaseStudies/a_blending_problem.html
import optflow as flow
x_1 = flow.Variable(cat=flow.continuous)
x_2 = flow.Variable(cat=flow.continuous)
cost_chicken = flow.Input(dtype=flow.float32)
cost_beef = flow.Input(dtype=flow.float32)
protein_lb = flow.Input(dtype=flow.float32)
fat_lb = flow.Input(dtype=flow.float32)
fibre_ub = flow.Input(dtype=flow.float32)
salt_ub = flow.Input(dtype=flow.float32)
obj = cost_chicken * x_1 + cost_beef * x_2
constraints = [
x_1 + x_2 == 100.0,
0.1 * x_1 + 0.2 * x_2 >= protein_lb,
0.08 * x_1 + 0.1 * x_2 >= fat_lb,
0.001 * x_1 + 0.005 * x_2 <= fibre_ub,
0.002 * x_1 + 0.005 * x_2 <= salt_ub
]
prob = flow.Problem(objective=obj, constraints=constraints, sense=flow.minimize)
opt_obj = prob.solve(solver=flow.programming, inputs={
cost_chicken: 0.013, cost_beef: 0.008, protein_lb: 8., fat_lb: 6., fibre_ub: 2., salt_ub: .4})
print(opt_obj)
print(x_1.optimized_value)
print(x_2.optimized_value)
[Run Online Demo] (password: )